In 2022, the Behavioural Insights Unit of India (BIU)–established through a tripartite agreement between NITI Aayog, the Bill and Melinda Gates Foundation, and the Centre for Social and Behaviour Change–undertook a study to understand how to scale empirically tested behavioural solutions that increase the take-up of iron and folic acid (IFA) tablets among pregnant women. For this we visited Jharkhand and Maharashtra, and our findings highlighted a blind spot that several researchers and policy practitioners interested in producing large-scale solutions miss: Was such an intervention designed to be scaled? A closer look revealed that the solutions were not ready for immediate implementation and required modifications in their design and delivery, which got us wondering why we failed to consider these aspects earlier.
Like us, quite a few researchers and practitioners interested in evidence-based policy implementation may have needed help with this question. Although evidence-based policymaking is widely backed, many of the tested solutions may not have the potential to be implemented at scale.
Why do solutions fail to scale?
Policy researchers often apply a scalability lens only after testing their proposed solution to establish causality. Unfortunately, evidence-based solutions are not frequently tested in real-life conditions. Therefore, an effective solution’s ability to yield consistent results upon scaling up remains unclear. The lack of a scalability lens might mean changes in the solution’s design or delivery at a later stage, which may compromise its expected impact. The Philadelphia government faced a similar issue during its attempt to scale up tested behavioural solutions to increase the enrolment of low-income senior citizens into a water discount programme. The solutions could not be scaled up as they were later concluded to be too expensive for the government. Although obvious in hindsight, the budget (or lack thereof) was not so obvious when the solution was being designed and tested as it was a factor that was controlled to establish causality between the intervention and the desired outcome.
In the short run, one tends to get consumed by the research question and may overlook the implementation aspects. This might increase the likelihood of failure when scaling up the solution, leaving one with wasted resources. In their paper on a behavioural design approach to public policy, Saugato Datta and Sendhil Mullainathan suggest that we need to move away from ‘boutique pilots’ that aim to test a specific insight and refocus efforts towards insights ‘built around the objective of achieving impact at scale’. Similarly, John List mentions that impact evaluation methodologies typically only focus on establishing causal relationships; however, one must also dissect why and how something is happening to assess whether the given solution can be scaled up.
Not only do we need to ensure the impact of the solution but we must also guarantee its sustainability.
Nonetheless, acknowledging that not all development research should focus solely on solving policy challenges is essential. Not all research questions are compatible with scale or are policy priorities. Scalability is a complex and dynamic puzzle to solve. Not only do we need to ensure the impact of the solution but we must also guarantee its sustainability. Adopting the scalability perspective throughout the solution’s life cycle—conceptualisation, design, testing, and implementation—is crucial. We must factor in the ground realities—the policy ecosystem, delivery system, and the contexts of the user and the delivery agent. How can this be done? Based on our lessons from the field, we present the following recommendations.
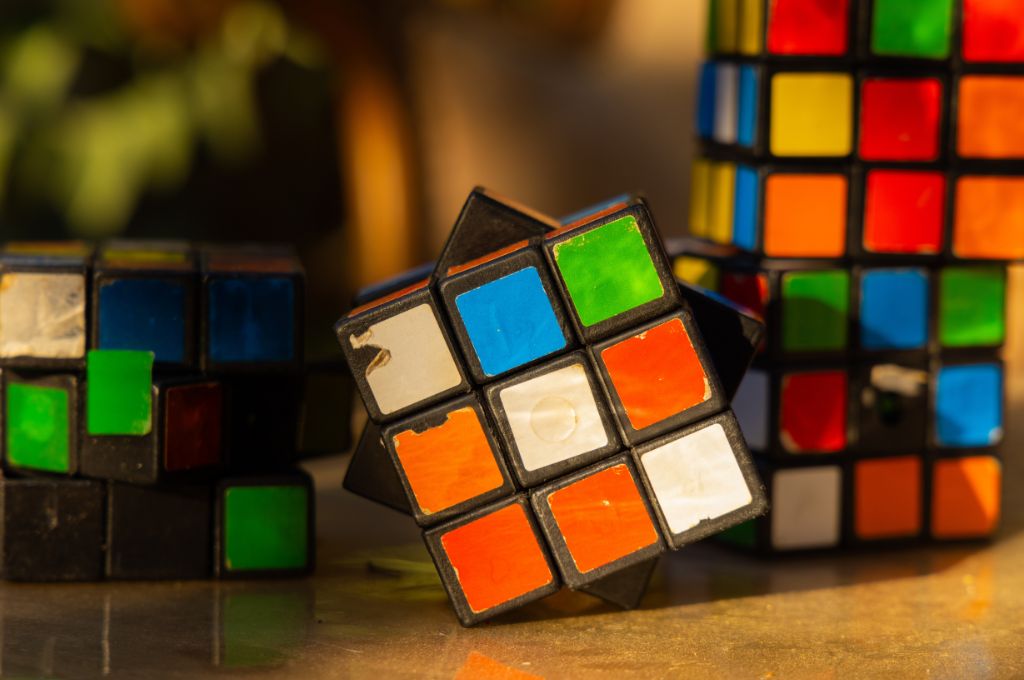
Solving for scale
1. Distilling the problem statement
At this stage, you want to take measures to ensure the sustainability of your solution. The first step is to distil and refine the problem statement based on policy priorities, focusing on what is a problem at scale so that you do not end up working on a solution that does not necessarily need to be scaled up. For example, we started with anaemia as a broad problem and narrowed it down to targeting anaemia in pregnant women, as more than 50 percent of them are anaemic in India.
Selecting the implementation pathway will provide you with a well-established ecosystem
Next, think about the implementation pathway. Will your solution be implemented by the government, by a nonprofit, or by the private sector? Selecting the implementation pathway will provide you with a well-established ecosystem to make the implementation process smoother. It also changes how you approach the problem. For example, we identified the government as our potential implementation partner and consequently had to figure out how our solutions could fit into this system. Had we chosen a nonprofit or a private organisation, our implementation pathway and delivery agents may have differed—changing the entire context of the solution.
After identifying the right partner, you may want to start aligning and collaborating with the relevant stakeholders on their priorities at the outset. This will not only ensure the sustainability of your solution but will also make the testing process more robust by enabling you to test in a realistic environment. For example, our solutions aimed to increase the uptake of IFA tablets among pregnant women were aligned with the government’s focus on the Anaemia Mukt Bharat strategy. Close alignment with key stakeholders ensures that your solution’s design speaks to the context, and that you are not reinventing the wheel.
All in all, you want to identify if your solution can be bundled with an existing ecosystem to give it some grounding and effectively utilise the implementation partner’s resources.
2. Creating a journey map
The next step will be to map the journey of your user within the chosen ecosystem to understand existing services and gaps. This involves identifying all the stakeholders—decision-makers, implementers, and delivery agents. As you design your solution and iterate on the prototype, the map will help you identify the expected barriers at every stage and resolve them ex-ante. In our project, we identified that capacity building for service delivery under large health and nutrition government programmes generally cascades from the national level down to the delivery agent, with each state leveraging its own training infrastructure and rules. These trainings may be conducted in large groups covering multiple topics or in smaller groups. Therefore, we anticipated that the training we recommend for implementing the solutions may be diluted due to the cognitive overload that the attendees of the training sessions may experience. To solve for the same, we printed delivery instructions at the back of the solutions for quick and easy reference.
Designing for scale
1. Leaving room for context
Scaling up successfully requires sustained effectiveness and generalisability across diverse populations. This means recognising the non-modifiable and modifiable features for the design and delivery of your solutions to suit the context (includingsociocultural and systemic differences) in which they will be applied. In our project, our visits to Jharkhand and Maharashtra helped us identify systemic differences in how their health departments function. As a result, we were able to distinguish aspects of our solutions that could be modified by the implementers to facilitate the implementation process. One such aspect was the language on the counselling card and calendar. Given the vast language diversity within and across Indian states, our final scale-up toolkit included open design files that allowed district or state officials to alter the language of the solutions as per local dialects.
2. Designing for the user and delivery system
The delivery of the solution is as important as the solution itself. The delivery agents’ motivations, mental bandwidth, and capabilities play a critical role in ensuring that interventions are delivered as planned. Similarly, budget availability, political priorities, and geographical variations also influence how the solution is delivered. We recognised that one of our solutions may be too expensive to be printed. We addressed this gap by allowing room for modifications in the way the solutions may be printed while retaining the behavioural features of the solutions.
Therefore, it is essential to be cognizant of the delivery ecosystem in order to identify and address potential pitfalls. For example, if you design a physical intervention such as a booklet, you must consider who will transport the booklets and how that would happen through the existing machinery.
Testing for scale
Lastly, it is important to test for scale and ensure the generalisability of the solution. Methods to ensure generalisability, such as testing on heterogeneous populations, are well documented. Most importantly, the solution must be tested realistically or within the identified ecosystem’s structure. For example, if you have identified anganwadi workers as the delivery agents, then they should deliver the solution during the testing phase as well. It would also be helpful to add a qualitative element to the study to capture details that quantitative instruments may not always record.
Scalability is not easy to account for. What works in one Indian state might not work in another. However, co-creating with the actual users—delivery agents, end users, and government agencies—can ensure that the final solution is more real-life compatible.
—
Know more
- Read this article on the factors that prevent programmes from scaling effectively.
- Learn about the insights acquired during the implementation of a livelihoods programme in Uttar Pradesh.